Publication Type
Journal Article
Version
publishedVersion
Publication Date
3-2007
Abstract
A new family of kernels is suggested for use in long run variance (LRV) estimation and robust regression testing. The kernels are constructed by taking powers of the Bartlett kernel and are intended to be used with no truncation (or bandwidth) parameter. As the power parameter ([rho]) increases, the kernels become very sharp at the origin and increasingly downweight values away from the origin, thereby achieving effects similar to a bandwidth parameter. Sharp origin kernels can be used in regression testing in much the same way as conventional kernels with no truncation, as suggested in the work of Kiefer and Vogelsang [2002a, Heteroskedasticity-autocorrelation robust testing using bandwidth equal to sample size. Econometric Theory 18, 1350-1366, 2002b, Heteroskedasticity-autocorrelation robust standard errors using the Bartlett kernel without truncation, Econometrica 70, 2093-2095] Analysis and simulations indicate that sharp origin kernels lead to tests with improved size properties relative to conventional tests and better power properties than other tests using Bartlett and other conventional kernels without truncation. If [rho] is passed to infinity with the sample size (T), the new kernels provide consistent LRV estimates. Within this new framework, untruncated kernel estimation can be regarded as a form of conventional kernel estimation in which the usual bandwidth parameter is replaced by a power parameter that serves to control the degree of downweighting. A data-driven method for selecting the power parameter is recommended for hypothesis testing. Simulations show that this method gives arise to a test with more accurate size than the conventional HAC t-test at the cost of a very small power loss.
Keywords
Heteroscedasticity and autocorrelation consistent standard error; Data-determined kernel estimation; Long run variance; Power parameter; Sharp origin kernel
Discipline
Econometrics
Research Areas
Econometrics
Publication
Journal of Statistical Planning and Inference
Volume
137
Issue
3
First Page
985
Last Page
1023
ISSN
0378-3758
Identifier
10.1016/j.jspi.2006.06.033
Publisher
Elsevier
Citation
PHILLIPS, Peter C. B.; SUN, Yixiao; and JIN, Sainan.
Long Run Variance Estimation and Robust Regression Testing Using Sharp Origin Kernels with No Truncation. (2007). Journal of Statistical Planning and Inference. 137, (3), 985-1023.
Available at: https://ink.library.smu.edu.sg/soe_research/318
Creative Commons License
This work is licensed under a Creative Commons Attribution-NonCommercial-No Derivative Works 4.0 International License.
Additional URL
https://doi.org/10.1016/j.jspi.2006.06.033
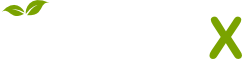
- Citations
- Citation Indexes: 28
- Policy Citations: 1
- Usage
- Downloads: 232
- Abstract Views: 72
- Captures
- Readers: 14