Publication Type
Journal Article
Version
submittedVersion
Publication Date
11-2016
Abstract
This paper provides a novel mechanism for identifying and estimating latent group structures in panel data using penalized techniques. We consider both linear and nonlinear models where the regression coefficients are heterogeneous across groups but homogeneous within a group and the group membership is unknown. Two approaches are consideredpenalized profile likelihood (PPL) estimation for the general nonlinear models without endogenous regressors, and penalized GMM (PGMM) estimation for linear models with endogeneity. In both cases, we develop a new variant of Lasso called classifier-Lasso (C-Lasso) that serves to shrink individual coefficients to the unknown group-specific coefficients. C-Lasso achieves simultaneous classification and consistent estimation in a single step and the classification exhibits the desirable property of uniform consistency. For PPL estimation, C-Lasso also achieves the oracle property so that group-specific parameter estimators are asymptotically equivalent to infeasible estimators that use individual group identity information. For PGMM estimation, the oracle property of C-Lasso is preserved in some special cases. Simulations demonstrate good finite-sample performance of the approach in both classification and estimation. Empirical applications to both linear and nonlinear models are presented.
Keywords
Classification, cluster analysis, dynamic panel, group Lasso, high dimensionality, nonlinear panel, oracle property, panel structure model, parameter heterogeneity, penalized least squares, penalized GMM, penalized profile likelihood
Discipline
Econometrics
Research Areas
Econometrics
Publication
Econometrica
Volume
84
Issue
6
First Page
2215
Last Page
2264
ISSN
0012-9682
Identifier
10.3982/ECTA12560
Publisher
Econometric Society
Citation
SU, Liangjun; SHI, Zhentao; and Peter C. B. PHILLIPS.
Identifying latent structures in panel data. (2016). Econometrica. 84, (6), 2215-2264.
Available at: https://ink.library.smu.edu.sg/soe_research/1911
Copyright Owner and License
Authors
Creative Commons License
This work is licensed under a Creative Commons Attribution-NonCommercial-No Derivative Works 4.0 International License.
Additional URL
https://doi.org/10.3982/ECTA12560
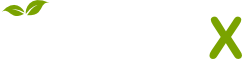
- Citations
- Citation Indexes: 147
- Policy Citations: 11
- Usage
- Downloads: 117
- Abstract Views: 85
- Captures
- Readers: 141
- Mentions
- Blog Mentions: 1