Publication Type
Journal Article
Version
publishedVersion
Publication Date
11-2024
Abstract
Ultra-high resolution image segmentation has raised increasing interests in recent years due to its realistic applications. In this paper, we innovate the widely used high-resolution image segmentation pipeline, in which an ultra-high resolution image is partitioned into regular patches for local segmentation and then the local results are merged into a high-resolution semantic mask. In particular, we introduce a novel locality-aware context fusion based segmentation model to process local patches, where the relevance between local patch and its various contexts are jointly and complementarily utilized to handle the semantic regions with large variations. Additionally, we present the alternating local enhancement module that restricts the negative impact of redundant information introduced from the contexts, and thus is endowed with the ability of fixing the locality-aware features to produce refined results. Furthermore, in comprehensive experiments, we demonstrate that our model outperforms other state-of-the-art methods in public benchmarks and verify the effectiveness of the proposed modules.
Keywords
Attention mechanisms, Context-guided vision model, Geo-spatial, Geo-spatial image segmentation, High resolution image segmentation, Images segmentations, Spatial images, Ultra-high resolution image segmentation, Ultrahigh resolution, Vision model
Discipline
Artificial Intelligence and Robotics | Databases and Information Systems
Research Areas
Data Science and Engineering; Information Systems and Management
Publication
International Journal of Computer Vision
Volume
132
Issue
11
First Page
5030
Last Page
5047
ISSN
0920-5691
Identifier
10.1007/s11263-024-02045-3
Publisher
Springer
Citation
LIU, Wenxi; LI, Qi; LIN, Xindai; YANG, Weixiang; HE, Shengfeng; and YU, Yuanlong.
Ultra-high resolution image segmentation via locality-aware context fusion and alternating local enhancement. (2024). International Journal of Computer Vision. 132, (11), 5030-5047.
Available at: https://ink.library.smu.edu.sg/sis_research/9770
Creative Commons License
This work is licensed under a Creative Commons Attribution-NonCommercial-No Derivative Works 4.0 International License.
Additional URL
https://doi.org/10.1007/s11263-024-02045-3
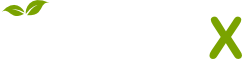
- Citations
- Citation Indexes: 2
- Usage
- Downloads: 1
- Abstract Views: 1
- Captures
- Readers: 2
- Mentions
- News Mentions: 1