Publication Type
Journal Article
Version
publishedVersion
Publication Date
12-2004
Abstract
Justification is an explanation that supports the verdict assigned to a claim in fact-checking. However, the task of justification generation is previously oversimplified as summarization of fact-check article authored by professional checkers. In this work, we propose a realistic approach to generate justification based on retrieved evidence. We present a new benchmark dataset called ExClaim for Explainable Claim verification, and introduce JustiLM, a novel few-shot retrieval-augmented language model to learn justification generation by leveraging fact-check articles as auxiliary resource during training. Our results show that JustiLM outperforms in-context learning (ICL)-enabled LMs including Flan-T5 and Llama2, and the retrieval-augmented model Atlas in few-shot setting. JustiLM also shows promising performance compared to GPT-4. Extending JustiLM for joint verdict prediction and justification generation improves verdict prediction with large margins.
Discipline
Artificial Intelligence and Robotics | Databases and Information Systems | Numerical Analysis and Scientific Computing
Research Areas
Data Science and Engineering
Publication
Transactions of the Association for Computational Linguistics
Volume
12
First Page
334
Last Page
354
ISSN
2307-387X
Identifier
10.1162/tacl_a_00649
Publisher
Massachusetts Institute of Technology Press
Citation
ZENG, Fengzhu and GAO, Wei.
JustiLM: Few-shot justification generation for explainable fact-checking of real-world claims. (2004). Transactions of the Association for Computational Linguistics. 12, 334-354.
Available at: https://ink.library.smu.edu.sg/sis_research/9441
Copyright Owner and License
Publisher
Creative Commons License
This work is licensed under a Creative Commons Attribution-NonCommercial-No Derivative Works 4.0 International License.
Additional URL
https://doi.org/10.1162/tacl_a_00649
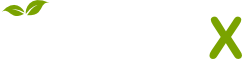
- Citations
- Citation Indexes: 5
- Usage
- Downloads: 9
- Abstract Views: 1
- Captures
- Readers: 17
- Mentions
- News Mentions: 1
Included in
Artificial Intelligence and Robotics Commons, Databases and Information Systems Commons, Numerical Analysis and Scientific Computing Commons