Publication Type
Conference Proceeding Article
Version
publishedVersion
Publication Date
8-2017
Abstract
In this paper, we propose a deep CNN to tackle the image restoration problem by learning the structured residual. Previous deep learning based methods directly learn the mapping from corrupted images to clean images, and may suffer from the gradient exploding/vanishing problems of deep neural networks. We propose to address the image restoration problem by learning the structured details and recovering the latent clean image together, from the shared information between the corrupted image and the latent image. In addition, instead of learning the pure difference (corruption), we propose to add a 'residual formatting layer' to format the residual to structured information, which allows the network to converge faster and boosts the performance. Furthermore, we propose a cross-level loss net to ensure both pixel-level accuracy and semantic-level visual quality. Evaluations on public datasets show that the proposed method outperforms existing approaches quantitatively and qualitatively.
Keywords
Computer vision, Deep learning, Deep neural networks, Pattern recognition, Restoration, Semantics
Discipline
Artificial Intelligence and Robotics | Graphics and Human Computer Interfaces
Research Areas
Software and Cyber-Physical Systems
Publication
Proceedings of the IEEE Conference on Computer Vision and Pattern Recognition Workshops (CVPRW), 2017, Honolulu, Hawaii, USA, July 21-26
First Page
1034
Last Page
1042
ISBN
9781538607336
Identifier
10.1109/CVPRW.2017.140
Publisher
IEEE
City or Country
New York, NY, USA
Citation
JIAO, Jianbo; TU, Wei-chih; and HE, Shengfeng.
FormResNet: Formatted residual learning for image restoration. (2017). Proceedings of the IEEE Conference on Computer Vision and Pattern Recognition Workshops (CVPRW), 2017, Honolulu, Hawaii, USA, July 21-26. 1034-1042.
Available at: https://ink.library.smu.edu.sg/sis_research/8428
Copyright Owner and License
Authors
Creative Commons License
This work is licensed under a Creative Commons Attribution-NonCommercial-No Derivative Works 4.0 International License.
Additional URL
https://doi.org/10.1109/CVPRW.2017.140
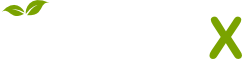
- Citations
- Patent Family Citations: 1
- Citation Indexes: 62
- Usage
- Downloads: 7
- Abstract Views: 3
- Captures
- Readers: 70
Included in
Artificial Intelligence and Robotics Commons, Graphics and Human Computer Interfaces Commons