Publication Type
Conference Proceeding Article
Version
publishedVersion
Publication Date
10-2017
Abstract
Subitizing (i.e., instant judgement on the number) and detection of salient objects are human inborn abilities. These two tasks influence each other in the human visual system. In this paper, we delve into the complementarity of these two tasks. We propose a multi-task deep neural network with weight prediction for salient object detection, where the parameters of an adaptive weight layer are dynamically determined by an auxiliary subitizing network. The numerical representation of salient objects is therefore embedded into the spatial representation. The proposed joint network can be trained end-to-end using backpropagation. Experiments show the proposed multi-task network outperforms existing multi-task architectures, and the auxiliary subitizing network provides strong guidance to salient object detection by reducing false positives and producing coherent saliency maps. Moreover, the proposed method is an unconstrained method able to handle images with/without salient objects. Finally, we show state-of-theart performance on different salient object datasets.
Keywords
Computer vision, Deep neural networks, Object recognition, Adaptive weights, False positive, Human visual system, Joint network, Numerical representation, Salient object detection, Salient objects, Spatial representations, Object detection
Discipline
Databases and Information Systems
Research Areas
Information Systems and Management
Publication
Proceedings of the IEEE International Conference on Computer Vision
First Page
1059
Last Page
1067
ISBN
9781538610329
Identifier
10.1109/ICCV.2017.120
Publisher
IEEE
City or Country
Italy
Citation
HE, Shengfeng; JIAO, Jianbo; ZHANG, Xiaodan; HAN, Guoqiang; and LAU, Rynson W.H.
Delving into salient object subitizing and detection. (2017). Proceedings of the IEEE International Conference on Computer Vision. 1059-1067.
Available at: https://ink.library.smu.edu.sg/sis_research/8424
Copyright Owner and License
Authors
Creative Commons License
This work is licensed under a Creative Commons Attribution-NonCommercial-No Derivative Works 4.0 International License.
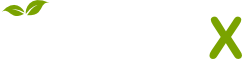
- Citations
- Citation Indexes: 53
- Patent Family Citations: 1
- Usage
- Downloads: 6
- Abstract Views: 1
- Captures
- Readers: 92