Stochastic economic lot scheduling via self-attention based deep reinforcement learning
Publication Type
Journal Article
Publication Date
2-2023
Abstract
The Stochastic Economic Lot Scheduling Problem (SELSP) is a difficult dynamic optimization problem with wide industrial applications. Traditional methods such as hyper-heuristics are manually designed based on substantial expert knowledge, which may limit their optimization performance. Recently, Deep Reinforcement Learning (DRL) is shown to be promising in automatically learning scheduling policies for SELSP. However, its performance is still quite far from that of hyper-heuristics, due to the lack of suitable deep models. In this paper, we propose a novel DRL method to learn dynamic scheduling policies for SELSP in an end-to-end fashion. Based on self-attention, our method can effectively extract useful features from raw state information, and is flexible in handling different numbers of products, which is not viable for previous methods. Experiments on a complex biopharmaceutical manufacturing process show that our method outperforms a recent DRL method and state-of-the-art hyper-heuristics. Moreover, the trained policy performs better in environments different from training with demand forecast errors and varying number of products, showing its strong robustness and generalization ability.Note to Practitioners-The Stochastic Economic Lot Scheduling Problem (SELSP) is an important problem for manufacturing enterprises, which is to optimally balance the production and inventory so as to minimize the total cost. However, SELSP is very challenging to solve due to the involvement of uncertain factors such as customer demands and machine failures. Traditional methods for solving SELSP, such as heuristic policies and hyper-heuristics, heavily rely on human experiences to design and hence the performance could be limited. This paper proposes a Deep Reinforcement Learning (DRL) based method to automatically learn scheduling policy for solving SELSP, which could alleviate the above limitation through a self-attention based feature extraction mechanism and reward based training. Experimental results on a realistic manufacturing process show that our method can deliver higher revenue than conventional manual policy and an existing DRL based method.
Keywords
Production, Job shop scheduling, Metaheuristics, Costs, Dynamic scheduling, Reinforcement learning, Deep learning, Deep reinforcement learning, Stochastic economic lot scheduling, Self-attention
Discipline
Operations Research, Systems Engineering and Industrial Engineering | Theory and Algorithms | Transportation
Research Areas
Intelligent Systems and Optimization
Publication
IEEE Transactions on Automation Science and Engineering
First Page
1
Last Page
12
ISSN
1545-5955
Identifier
10.1109/TASE.2023.3248229
Publisher
Institute of Electrical and Electronics Engineers
Citation
SONG, Wen; MI, Nan; LI, Qiqiang; ZHUANG, Jing; and CAO, Zhiguang.
Stochastic economic lot scheduling via self-attention based deep reinforcement learning. (2023). IEEE Transactions on Automation Science and Engineering. 1-12.
Available at: https://ink.library.smu.edu.sg/sis_research/8201
Additional URL
http://doi.org/10.1109/TASE.2023.3248229
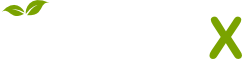
- Citations
- Citation Indexes: 8
- Usage
- Abstract Views: 9
- Captures
- Readers: 29
- Mentions
- News Mentions: 2