Identifiable, but not visible: A privacy-preserving person reidentfication scheme
Publication Type
Journal Article
Publication Date
4-2023
Abstract
Person re-identification (Person Re-ID) is widely regarded as a promising technique to identify a target person through surveillance cameras in the wild. Nevertheless, person Re-ID leads to severe personal image privacy concerns as personal images are stipulated by laws and guidelines as private data. To address these concerns, this article explores the first solution for building a privacy-preserving person Re-ID system. Specifically, this article formulizes privacy-preserving person Re-ID as similarity metrics of encrypted feature vectors because the underlying operation of person Re-ID is to compute the similarity of feature vectors that are extracted from person images by a machine learning model. However, feature vectors are generally denoted by floating-point numbers. To this end, this article exploits a series of new encoding mechanisms and secure batch computing protocols to encrypt floating-point feature vectors and achieve the underlying operation of person Re-ID. Rigorous theoretical analyses demonstrate that this work achieves person Re-ID without compromising any personal image privacy. Furthermore, the proposed secure batch protocols significantly enhance the performance of privacy-preserving person Re-ID while outputting the same precision as the previous method.
Keywords
Batch computation, image privacy, person Re-ID, privacy-preserving identification, secure computing
Discipline
Databases and Information Systems
Research Areas
Data Science and Engineering
Publication
IEEE Transactions on Reliability
First Page
1
Last Page
13
ISSN
0018-9529
Identifier
10.1109/TR.2023.3258983
Publisher
Institute of Electrical and Electronics Engineers
Citation
ZHAO, Bowen; LI, Yingjiu; LIU, Ximeng; LI, Xiaoguo; PANG, Hwee Hwa; and DENG, Robert H..
Identifiable, but not visible: A privacy-preserving person reidentfication scheme. (2023). IEEE Transactions on Reliability. 1-13.
Available at: https://ink.library.smu.edu.sg/sis_research/8112
Additional URL
https://doi.org/10.1109/TR.2023.3258983
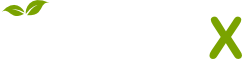
- Citations
- Citation Indexes: 3
- Usage
- Abstract Views: 4
- Captures
- Readers: 8
- Mentions
- News Mentions: 1