Publication Type
Journal Article
Version
publishedVersion
Publication Date
5-2016
Abstract
Current methods of dental identification are mainly based on 2D dental radiographs which suffer from speed and accuracy limitations. In this paper, we present an efficient dental identification approach based on 3D dental models. We propose a novel shape descriptor, the Signed Feature Histogram (SFH), which is highly discriminative and can be easily computed to describe the local surface. Based on the SFH, a learning keypoint detection method is adopted to accurately detect the desired keypoints on both antemortem (AM) and postmortem (PM) models. For a given PM model, the optimal initial alignment to the AM model to be matched can be found efficiently and robustly by matching the SFHs between the keypoints. The final matching score is obtained by running the iterative closest point algorithm which further refines the initial alignment. We have performed comparative experiments for the SFH on a public dataset, and state-of-the-art performance is achieved. We also test the identification method on a database of 200 AM models and tested the performance of the proposed approach on 3 different PM datasets comprising complete, incomplete and single tooth models respectively. The experimental results show that both high accuracy and efficiency are achieved with 100% Rank-1 identification accuracy on both complete and incomplete PM models and 74% Rank-1 accuracy on single tooth PM models. The running time is only 300 s on average which is about 80 times faster than many 2D methods which can take several hours to identify one subject.
Keywords
Dental biometrics, Tooth recognition, Postmortem identification, Shape descriptor, Keypoint detection, Shape matching, Random Forest
Discipline
Artificial Intelligence and Robotics | Graphics and Human Computer Interfaces
Research Areas
Information Systems and Management
Publication
Pattern Recognition
Volume
60
First Page
189
Last Page
204
ISSN
0031-3203
Identifier
10.1016/j.patcog.2016.05.007
Publisher
Elsevier
Citation
ZHANG, Zhiyuan; ONG, Sim Heng; ZHONG, Xin; and FOONG, Kelvin W. C..
Efficient 3D dental identification via signed feature histogram and learning keypoint detection. (2016). Pattern Recognition. 60, 189-204.
Available at: https://ink.library.smu.edu.sg/sis_research/7940
Creative Commons License
This work is licensed under a Creative Commons Attribution-NonCommercial-No Derivative Works 4.0 International License.
Additional URL
https://doi.org/10.1016/j.patcog.2016.05.007
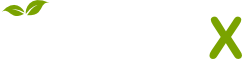
- Citations
- Citation Indexes: 15
- Patent Family Citations: 1
- Usage
- Downloads: 68
- Abstract Views: 6
- Captures
- Readers: 37
Included in
Artificial Intelligence and Robotics Commons, Graphics and Human Computer Interfaces Commons