Publication Type
Journal Article
Version
publishedVersion
Publication Date
4-2021
Abstract
Peak Response Map (PRM) highlighting the discriminative regions can be extracted from a pre-trained classification network. We can accurately localize instances of each class with the help of these response maps. However, these maps cannot provide reliable information for segmentation even with off-the-shelf object proposals. This is because neither PRM nor the proposals know which regions can be regarded as a complete instance. In this paper, we tackle this problem by proposing an Instance-aware Cue propagation Network (ICN) with a new proposal-matching strategy. In particular, the ICN aims to filter out background distractions and cover the complete instance, while our proposed proposal-matching strategy adds a re-balancing constraint on the contributions of multi-scale object proposals. Extensive experiments conducted on the PASCAL VOC 2012 dataset show the superior performance of our method over weakly-supervised state-of-the-arts for both semantic and instance segmentation.(c) 2021 Elsevier B.V. All rights reserved.
Keywords
Weakly supervised learning, Instance segmentation
Discipline
Information Security
Research Areas
Information Systems and Management
Publication
Neurocomputing
Volume
447
First Page
1
Last Page
9
ISSN
0925-2312
Identifier
10.1016/j.neucom.2021.02.093
Publisher
Elsevier
Citation
XIN, Huang; ZHU, Qianshu; LIU, Yongtuo; and HE, Shengfeng.
Weakly supervised segmentation via instance-aware propagation. (2021). Neurocomputing. 447, 1-9.
Available at: https://ink.library.smu.edu.sg/sis_research/7843
Creative Commons License
This work is licensed under a Creative Commons Attribution-NonCommercial-No Derivative Works 4.0 International License.
Additional URL
https://doi.org/10.1016/j.neucom.2021.02.093
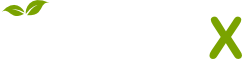
- Citations
- Citation Indexes: 3
- Patent Family Citations: 1
- Usage
- Downloads: 15
- Abstract Views: 6
- Captures
- Readers: 7