Publication Type
Journal Article
Version
acceptedVersion
Publication Date
3-2023
Abstract
Energy costs represent a significant share of the total cost of ownership in high performance computing (HPC) systems. Using a unique data set collected by massive sensor networks in a peta scale national supercomputing center, we first present an explanatory model to identify key factors that affect energy consumption in supercomputing. Our analytic results show that, not only does computing node utilization significantly affect energy consumption, workload distribution among the nodes also has significant effects and could effectively be leveraged to improve energy efficiency. Next, we establish the high model performance using in-sample and out-of-sample analyses. We then develop prescriptive models for energy-optimal runtime workload management and extend the models to consider energy consumption and job performance tradeoffs. Specifically, we present four dynamic resource management methodologies (packing, load balancing, threshold-based switching, and energy optimization), model their application at two levels (purely within-rack and jointly cross-rack resource allocation), and explore runtime resource redistribution policies for jobs under the emergent principle of computational steering and comparatively evaluate strategies that use computational steering with those that do not. Our experimental studies show that packing is preferred when the total workload of a rack is higher than a threshold and load balancing is preferred when it is lower. These results lead to a threshold strategy that yields near-optimal energy efficiency under all workload conditions. We further calibrate the energy-optimal resource allocations over the full range of workloads and present a bicriteria evaluation to consider energy consumption and job performance tradeoffs. We demonstrate significant energy savings of our proposed strategies under various workload conditions. We conclude with implementation guidelines and policy insights into energy efficient computing resource management in large supercomputing data centers.
Keywords
high-performance computing, data center, energy-efficient operation, data analytics, autoregressive model, dynamic panel data, optimization
Discipline
Databases and Information Systems | Numerical Analysis and Scientific Computing
Research Areas
Information Systems and Management
Publication
Information Systems Research
Volume
34
Issue
4
First Page
1664
Last Page
1685
ISSN
1047-7047
Identifier
10.1287/isre.2023.1208
Publisher
Institute for Operations Research and Management Sciences
Citation
GUO, Zhiling; LI, Jin; and RAMESH, Ram.
Green data analytics of supercomputing from massive sensor networks: Does workload distribution matter?. (2023). Information Systems Research. 34, (4), 1664-1685.
Available at: https://ink.library.smu.edu.sg/sis_research/7813
Copyright Owner and License
Authors
Creative Commons License
This work is licensed under a Creative Commons Attribution-NonCommercial-No Derivative Works 4.0 International License.
Additional URL
https://doi.org/10.1287/isre.2023.1208
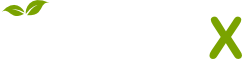
- Citations
- Citation Indexes: 2
- Usage
- Downloads: 288
- Abstract Views: 18
- Captures
- Readers: 43
- Mentions
- News Mentions: 2
Included in
Databases and Information Systems Commons, Numerical Analysis and Scientific Computing Commons