Publication Type
Journal Article
Version
publishedVersion
Publication Date
5-2022
Abstract
Machine learning is disruptive. At the same time, machine learning can only succeed by collaboration among many parties in multiple steps naturally as pipelines in an eco-system, such as collecting data for possible machine learning applications, collaboratively training models by multiple parties and delivering machine learning services to end users. Data are critical and penetrating in the whole machine learning pipelines. As machine learning pipelines involve many parties and, in order to be successful, have to form a constructive and dynamic eco-system, marketplaces and data pricing are fundamental in connecting and facilitating those many parties. In this article, we survey the principles and the latest research development of data pricing in machine learning pipelines. We start with a brief review of data marketplaces and pricing desiderata. Then, we focus on pricing in three important steps in machine learning pipelines. To understand pricing in the step of training data collection, we review pricing raw data sets and data labels. We also investigate pricing in the step of collaborative training of machine learning models and overview pricing machine learning models for end users in the step of machine learning deployment. We also discuss a series of possible future directions.
Keywords
Data pricing, Data asset, Data governance
Discipline
Databases and Information Systems
Research Areas
Data Science and Engineering
Publication
Knowledge and Information Systems
Volume
64
Issue
6
First Page
1417
Last Page
1455
ISSN
0219-1377
Identifier
10.1007/s10115-022-01679-4
Publisher
Springer Verlag (Germany)
Citation
CONG, Zicun; LUO, Xuan; PEI, Jian; ZHU, Feida; and ZHANG, Yong.
Data pricing in machine learning pipelines. (2022). Knowledge and Information Systems. 64, (6), 1417-1455.
Available at: https://ink.library.smu.edu.sg/sis_research/7755
Creative Commons License
This work is licensed under a Creative Commons Attribution-NonCommercial-No Derivative Works 4.0 International License.
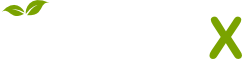
- Citations
- Citation Indexes: 30
- Patent Family Citations: 1
- Usage
- Downloads: 38
- Abstract Views: 12
- Captures
- Readers: 51