Publication Type
Conference Proceeding Article
Version
publishedVersion
Publication Date
10-2018
Abstract
Deep learning methods have been successfully applied to fashion retrieval. However, the latent meaning of learned feature vectors hinders the explanation of retrieval results and integration of user feedback. Fortunately, there are many online shopping websites organizing fashion items into hierarchical structures based on product taxonomy and domain knowledge. Such structures help to reveal how human perceive the relatedness among fashion products. Nevertheless, incorporating structural knowledge for deep learning remains a challenging problem. This paper presents techniques for organizing and utilizing the fashion hierarchies in deep learning to facilitate the reasoning of search results and user intent. The novelty of our work originates from the development of an EI (Exclusive & Independent) tree that can cooperate with deep models for end-to-end multimodal learning. EI tree organizes the fashion concepts into multiple semantic levels and augments the tree structure with exclusive as well as independent constraints. It describes the different relationships among sibling concepts and guides the end-to-end learning of multi-level fashion semantics. From EI tree, we learn an explicit hierarchical similarity function to characterize the semantic similarities among fashion products. It facilitates the interpretable retrieval scheme that can integrate the concept-level feedback. Experiment results on two large fashion datasets show that the proposed approach can characterize the semantic similarities among fashion items accurately and capture user's search intent precisely, leading to more accurate search results as compared to the state-of-the-art methods.
Keywords
Attribute manipulation, EI tree, Multimodal fashion retrieval
Discipline
Artificial Intelligence and Robotics | Databases and Information Systems
Research Areas
Data Science and Engineering; Intelligent Systems and Optimization
Publication
MM '18: Proceedings of the 26th ACM international conference on Multimedia
First Page
1571
Last Page
1579
ISBN
9781450356657
Identifier
10.1145/3240508.3240646
Publisher
Association for Computing Machinery
City or Country
New York, NY, United States
Citation
LIAO, Lizi; HE, Xiangnan; ZHAO, Bo; NGO, Chong-Wah; and CHUA, Tat-Seng.
Interpretable multimodal retrieval for fashion products. (2018). MM '18: Proceedings of the 26th ACM international conference on Multimedia. 1571-1579.
Available at: https://ink.library.smu.edu.sg/sis_research/7677
Creative Commons License
This work is licensed under a Creative Commons Attribution-NonCommercial-No Derivative Works 4.0 International License.
Additional URL
https://doi.org/10.1145/3240508.3240646
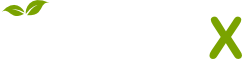
- Citations
- Citation Indexes: 60
- Patent Family Citations: 1
- Usage
- Downloads: 183
- Abstract Views: 37
- Captures
- Readers: 96