Publication Type
Journal Article
Version
publishedVersion
Publication Date
1-2012
Abstract
Text categorization is a significant tool to manage and organize the surging text data. Many text categorization algorithms have been explored in previous literatures, such as KNN, Naive Bayes and Support Vector Machine. KNN text categorization is an effective but less efficient classification method. In this paper, we propose an improved KNN algorithm for text categorization, which builds the classification model by combining constrained one pass clustering algorithm and KNN text categorization. Empirical results on three benchmark corpora show that our algorithm can reduce the text similarity computation substantially and outperform the-state-of-the-art KNN, Naive Bayes and Support Vector Machine classifiers. In addition, the classification model constructed by the proposed algorithm can be updated incrementally, and it has great scalability in many real-word applications. (C) 2011 Elsevier Ltd. All rights reserved.
Keywords
Text categorization, KNN text categorization, One-pass clustering, Spam filtering
Discipline
Databases and Information Systems | Theory and Algorithms
Research Areas
Data Science and Engineering
Publication
Expert Systems with Applications
Volume
39
Issue
1
First Page
1503
Last Page
1509
ISSN
0957-4174
Identifier
10.1016/j.eswa.2011.08.040
Publisher
Elsevier
Citation
JIANG, Shengyi; PANG, Guansong; WU, Meiling; and KUANG, Limin.
An improved K-nearest-neighbor algorithm for text categorization. (2012). Expert Systems with Applications. 39, (1), 1503-1509.
Available at: https://ink.library.smu.edu.sg/sis_research/7542
Creative Commons License
This work is licensed under a Creative Commons Attribution-NonCommercial-No Derivative Works 4.0 International License.
Additional URL
http://doi.org/10.1016/j.eswa.2011.08.040
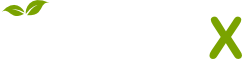
- Citations
- Citation Indexes: 243
- Usage
- Downloads: 507
- Abstract Views: 18
- Captures
- Readers: 366
- Mentions
- News Mentions: 1