Publication Type
Conference Proceeding Article
Version
publishedVersion
Publication Date
3-2022
Abstract
Artificial intelligence (AI) and machine learning (ML) algorithms are increasingly being explored to support various decision-making tasks in health (e.g. rehabilitation assessment). However, the development of such AI/ML-based decision support systems is challenging due to the expensive process to collect an annotated dataset. In this paper, we describe the development process of a human-AI collaborative, clinical decision support system that augments an ML model with a rule-based (RB) model from domain experts. We conducted its empirical evaluation in the context of assessing physical stroke rehabilitation with the dataset of three exercises from 15 post-stroke survivors and therapists. Our results bring new insights on the efficient development and annotations of a decision support system: when an annotated dataset is not available initially, the RB model can be used to assess post-stroke survivor’s quality of motion and identify samples with low confidence scores to support efficient annotations for training an ML model. Specifically, our system requires only 22 - 33% of annotations from therapists to train an ML model that achieves equally good performance with an ML model with all annotations from a therapist. Our work discusses the values of a human-AI collaborative approach for effectively collecting an annotated dataset and supporting a complex decision-making task.
Keywords
Human Centered AI, Human-AI Collaboration, Human-In-the-Loop Systems, Clinical Decision Support Systems, Physical Stroke Rehabilitation Assessment
Discipline
Artificial Intelligence and Robotics
Research Areas
Intelligent Systems and Optimization
Publication
Proceedings of the 27th International Conference on Intelligent User Interfaces, Helsinki, Finland, March 22-25
First Page
4
Last Page
14
ISBN
9781450391443
Identifier
10.1145/3490099.3511112
Publisher
ACM
City or Country
New York, NY, USA
Citation
LEE, Min Hun; SIEWIOREK, Daniel P.; SMAILAGIC, Asim; BERNARDINO, Alexandre; and I BADIA, Sergi Bermúdez.
Towards efficient annotations for a human-AI collaborative, clinical decision support system: A case study on physical stroke rehabilitation assessment. (2022). Proceedings of the 27th International Conference on Intelligent User Interfaces, Helsinki, Finland, March 22-25. 4-14.
Available at: https://ink.library.smu.edu.sg/sis_research/7307
Creative Commons License
This work is licensed under a Creative Commons Attribution-NonCommercial-No Derivative Works 4.0 International License.
Additional URL
http://doi.org/10.1145/3490099.3511112
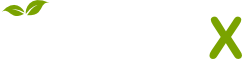
- Citations
- Citation Indexes: 9
- Usage
- Downloads: 25
- Abstract Views: 24
- Captures
- Readers: 25
- Mentions
- Blog Mentions: 1