Publication Type
Conference Proceeding Article
Version
acceptedVersion
Publication Date
5-2021
Abstract
Accurate, real-time object detection on resource-constrained devices enables autonomous mobile vision applications such as traffic surveillance, situational awareness, and safety inspection, where it is crucial to detect both small and large objects in crowded scenes. Prior studies either perform object detection locally on-board or offload the task to the edge/cloud. Local object detection yields low accuracy on small objects since it operates on low-resolution videos to fit in mobile memory. Offloaded object detection incurs high latency due to uploading high-resolution videos to the edge/cloud. Rather than either pure local processing or offloading, we propose to detect large objects locally while offloading small object detection to the edge. The key challenge is to reduce the latency of small object detection. Accordingly, we develop EdgeDuet, the first edge-device collaborative framework for enhancing small object detection with tile-level parallelism. It optimizes the offloaded detection pipeline in tiles rather than the entire frame for high accuracy and low latency. Evaluations on drone vision datasets under LTE, WiFi 2.4GHz, WiFi 5GHz show that EdgeDuet outperforms local object detection in small object detection accuracy by 233.0%. It also improves the detection accuracy by 44.7% and latency by 34.2% over the state-of-the-art offloading schemes.
Discipline
Artificial Intelligence and Robotics | Graphics and Human Computer Interfaces
Research Areas
Software and Cyber-Physical Systems
Publication
Proceedings of the IEEE International Conference on Computer Communications
Identifier
10.1109/INFOCOM42981.2021.9488843
City or Country
Vancouver, BC, Canada
Citation
WANG, Xu; YANG, Zheng; WU, Jiahang; ZHAO, Yi; and ZHOU, Zimu.
Edgeduet: Tiling small object detection for edge assisted autonomous mobile vision. (2021). Proceedings of the IEEE International Conference on Computer Communications.
Available at: https://ink.library.smu.edu.sg/sis_research/6747
Creative Commons License
This work is licensed under a Creative Commons Attribution-NonCommercial-No Derivative Works 4.0 International License.
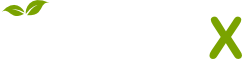
- Citations
- Citation Indexes: 42
- Patent Family Citations: 1
- Usage
- Downloads: 444
- Abstract Views: 52
- Captures
- Readers: 24
Included in
Artificial Intelligence and Robotics Commons, Graphics and Human Computer Interfaces Commons