Publication Type
Journal Article
Version
acceptedVersion
Publication Date
5-2023
Abstract
Future mobile devices are anticipated to perceive, understand and react to the world on their own by running multiple correlated deep neural networks locally on-device. Yet the complexity of these deep models needs to be trimmed down both within-model and cross-model to fit in mobile storage and memory. Previous studies squeeze the redundancy within a single model. In this work, we aim to reduce the redundancy across multiple models. We propose Multi-Task Zipping (MTZ), a framework to automatically merge correlated, pre-trained deep neural networks for cross-model compression. Central in MTZ is a layer-wise neuron sharing and incoming weight updating scheme that induces a minimal change in the error function. MTZ inherits information from each model and demands light retraining to re-boost the accuracy of individual tasks. MTZ supports typical network layers (fully-connected, convolutional and residual) and applies to inference tasks with different input domains. Evaluations show that MTZ can fully merge the hidden layers of two VGG-16 networks with a 3.18% increase in the test error averaged on ImageNet for object classification and CelebA for facial attribute classification, or share 39.61% parameters between the two networks with
Keywords
Deep Neural Networks, Model Compression, Multi-Task Learning
Discipline
OS and Networks | Software Engineering
Research Areas
Software and Cyber-Physical Systems
Publication
IEEE Transactions on Mobile Computing
Volume
22
Issue
5
First Page
2878
Last Page
2891
ISSN
1536-1233
Identifier
10.1109/TMC.2021.3124306
Publisher
Institute of Electrical and Electronics Engineers
Citation
HE, Xiaoxi; WANG, Xu; ZHOU, Zimu; WU, Jiahang; YANG, Zheng; and THIELE, Lothar.
On-device deep multi-task inference via multi-task zipping. (2023). IEEE Transactions on Mobile Computing. 22, (5), 2878-2891.
Available at: https://ink.library.smu.edu.sg/sis_research/6724
Copyright Owner and License
Authors
Creative Commons License
This work is licensed under a Creative Commons Attribution-NonCommercial-No Derivative Works 4.0 International License.
Additional URL
https://doi.org/10.1109/TMC.2021.3124306
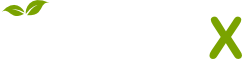
- Citations
- Citation Indexes: 6
- Usage
- Downloads: 172
- Abstract Views: 22
- Captures
- Readers: 8
- Mentions
- News Mentions: 1