Publication Type
Journal Article
Version
acceptedVersion
Publication Date
10-2022
Abstract
Machine learning's grand ambition is the mathematical modeling of reality. The recent years have seen major advances using deep-learned techniques that model reality implicitly; however, corresponding advances in explicit mathematical models have been noticeably lacking. We believe this dichotomy is rooted in the limitations of the current statistical tools, which struggle to make sense of the high dimensional generative processes that natural data seems to originate from. This paper proposes a new, distance based statistical technique which allows us to develop elegant mathematical models of such generative processes. Our model suggests that each semantic concept has an associated distinctive-shell which encapsulates almost-all instances of itself and excludes almost-all others. creating the first, explicit mathematical representation of the constraints which make machine learning possible.
Keywords
Semantics, Mathematical model, Machine learning, Random variables, Manifolds, Machine learning algorithms, Prediction algorithms
Discipline
Artificial Intelligence and Robotics
Research Areas
Data Science and Engineering
Publication
IEEE Transactions on Pattern Analysis and Machine Intelligence
Volume
44
Issue
10
First Page
6438
Last Page
6453
ISSN
0162-8828
Identifier
10.1109/TPAMI.2021.3084598
Publisher
Institute of Electrical and Electronics Engineers
Citation
LIN, Wen-yan; LIU, Siying; REN, Changhao; CHEUNG, Ngai-Man; LI, Hongdong; and MATSUSHITA, Yasuyuki.
Shell theory: A statistical model of reality. (2022). IEEE Transactions on Pattern Analysis and Machine Intelligence. 44, (10), 6438-6453.
Available at: https://ink.library.smu.edu.sg/sis_research/6222
Copyright Owner and License
Authors
Creative Commons License
This work is licensed under a Creative Commons Attribution-NonCommercial-No Derivative Works 4.0 International License.
Additional URL
https://doi.org/10.1109/TPAMI.2021.3084598
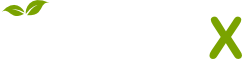
- Citations
- Citation Indexes: 3
- Usage
- Downloads: 349
- Abstract Views: 37
- Captures
- Readers: 13
- Mentions
- References: 2