Publication Type
Conference Proceeding Article
Version
publishedVersion
Publication Date
7-2009
Abstract
Nearest-neighbor collaborative filtering provides a successful means of generating recommendations for web users. However, this approach suffers from several shortcomings, including data sparsity and noise, the cold-start problem, and scalability. In this work, we present a novel method for recommending items to users based on expert opinions. Our method is a variation of traditional collaborative filtering: rather than applying a nearest neighbor algorithm to the user-rating data, predictions are computed using a set of expert neighbors from an independent dataset, whose opinions are weighted according to their similarity to the user. This method promises to address some of the weaknesses in traditional collaborative filtering, while maintaining comparable accuracy. We validate our approach by predicting a subset of the Netflix data set. We use ratings crawled from a web portal of expert reviews, measuring results both in terms of prediction accuracy and recommendation list precision. Finally, we explore the ability of our method to generate useful recommendations, by reporting the results of a user-study where users prefer the recommendations generated by our approach.
Keywords
Recommender Systems, Collaborative Filtering, Experts, Cosine Similarity, Nearest Neighbors, Top-N Recommendations
Discipline
Numerical Analysis and Scientific Computing | Theory and Algorithms
Research Areas
Data Science and Engineering; Intelligent Systems and Optimization
Publication
Proceedings of the 32nd Annual International ACM SIGIR Conference on Research and Development in Information Retrieval, SIGIR 2009, Boston, MA, United States, July 19-23
First Page
532
Last Page
539
ISBN
978-1-60558-483-6
Identifier
10.1145/1571941.1572033
Publisher
ACM
City or Country
New York
Citation
1
Creative Commons License
This work is licensed under a Creative Commons Attribution-NonCommercial-No Derivative Works 4.0 International License.
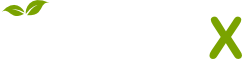
- Citations
- Citation Indexes: 127
- Usage
- Downloads: 125
- Abstract Views: 8
- Captures
- Readers: 134
- Mentions
- Blog Mentions: 2