Publication Type
Journal Article
Version
acceptedVersion
Publication Date
6-2021
Abstract
With superhuman-level performance of face recognition, we are more concerned about the recognition of fine-grained attributes, such as emotion, age, and gender. However, given that the label space is extremely large and follows a long-tail distribution, it is quite expensive to collect sufficient samples for fine-grained attributes. This results in imbalanced training samples and inferior attribute recognition models. To this end, we propose the use of arbitrary attribute combinations, without human effort, to synthesize face images. In particular, to bridge the semantic gap between high-level attribute label space and low-level face image, we propose a novel neural-network-based approach that maps the target attribute labels to an embedding vector, which can be fed into a pretrained image decoder to synthesize a new face image. Furthermore, to regularize the attribute for image synthesis, we propose to use a perceptual loss to make the new image explicitly faithful to target attributes. Experimental results show that our approach can generate photorealistic face images from attribute labels, and more importantly, by serving as augmented training samples, these images can significantly boost the performance of attribute recognition model. The code is open-sourced at this link.
Keywords
Face, Face recognition, Image recognition, Image reconstruction, Task analysis, Gallium nitride, Decoding
Discipline
Graphics and Human Computer Interfaces
Research Areas
Data Science and Engineering
Publication
IEEE Transactions on Neural Networks and Learning Systems
Volume
32
Issue
6
First Page
2733
Last Page
2743
ISSN
2162-2388
Identifier
10.1109/TNNLS.2020.3007790
Publisher
Institute of Electrical and Electronics Engineers
Citation
LIU, Yaoyao; SUN, Qianru; HE Xiangnan; LIU An-An; SU Yuting; and CHUA Tat-Seng.
Generating face images with attributes for free. (2021). IEEE Transactions on Neural Networks and Learning Systems. 32, (6), 2733-2743.
Available at: https://ink.library.smu.edu.sg/sis_research/5643
Copyright Owner and License
Authors
Additional URL
https://doi.org/10.1109/TNNLS.2020.3007790
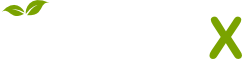
- Citations
- Citation Indexes: 6
- Usage
- Downloads: 58
- Abstract Views: 12
- Captures
- Readers: 12
- Mentions
- Blog Mentions: 1