Publication Type
Conference Proceeding Article
Version
publishedVersion
Publication Date
5-2020
Abstract
Software Composition Analysis (SCA) has gained traction in recent years with a number of commercial offerings from various companies. SCA involves vulnerability curation process where a group of security researchers, using various data sources, populate a database of open-source library vulnerabilities, which is used by a scanner to inform the end users of vulnerable libraries used by their applications. One of the data sources used is the National Vulnerability Database (NVD). The key challenge faced by the security researchers here is in figuring out which libraries are related to each of the reported vulnerability in NVD. In this article, we report our design and implementation of a machine learning system to help identify the libraries related to each vulnerability in NVD. The problem is that of extreme multi-label learning (XML), and we developed our system using the state-of-the-art FastXML algorithm. Our system is iteratively executed, improving the performance of the model over time. At the time of writing, it achieves F1@1 score of 0.53 with average F1@k score for k = 1, 2, 3 of 0.51 (F1@k is the harmonic mean of precision@k and recall@k). It has been deployed in Veracode as part of a machine learning system that helps the security researchers identify the likelihood of web data items to be vulnerability-related. In addition, we present evaluation results of our feature engineering and the FastXML tree number used. Our work formulates for the first time library name identification from NVD data as XML and it is also the first attempt at solving it in a complete production system.
Keywords
application security, open source software, machine learning, classifiers ensemble, self training
Discipline
Software Engineering
Research Areas
Software and Cyber-Physical Systems
Publication
ICSE '20: Proceedings of the 42nd ACM/IEEE International Conference on Software Engineering: 24 June - 16 July, Seoul, Virtual
First Page
90
Last Page
99
ISBN
9781450371230
Identifier
10.1145/3377813.3381360
Publisher
ACM
City or Country
New York
Citation
YANG, Chen; SANTOSA, Andrew; SHARMA, Asankhaya; and LO, David.
Automated identification of libraries from vulnerability data. (2020). ICSE '20: Proceedings of the 42nd ACM/IEEE International Conference on Software Engineering: 24 June - 16 July, Seoul, Virtual. 90-99.
Available at: https://ink.library.smu.edu.sg/sis_research/5501
Copyright Owner and License
Publisher
Creative Commons License
This work is licensed under a Creative Commons Attribution-NonCommercial-No Derivative Works 4.0 International License.
Additional URL
https://doi.org/10.1145/3377813.3381360
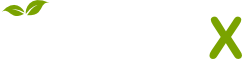
- Citations
- Citation Indexes: 19
- Usage
- Downloads: 614
- Abstract Views: 38
- Captures
- Readers: 40
- Mentions
- References: 2