Publication Type
Journal Article
Version
acceptedVersion
Publication Date
5-2015
Abstract
The use of domain knowledge in learning systems is expected to improve learning efficiency and reduce model complexity. However, due to the incompatibility with knowledge structure of the learning systems and real-time exploratory nature of reinforcement learning (RL), domain knowledge cannot be inserted directly. In this paper, we show how self-organizing neural networks designed for online and incremental adaptation can integrate domain knowledge and RL. Specifically, symbol-based domain knowledge is translated into numeric patterns before inserting into the self-organizing neural networks. To ensure effective use of domain knowledge, we present an analysis of how the inserted knowledge is used by the self-organizing neural networks during RL. To this end, we propose a vigilance adaptation and greedy exploitation strategy to maximize exploitation of the inserted domain knowledge while retaining the plasticity of learning and using new knowledge. Our experimental results based on the pursuit-evasion and minefield navigation problem domains show that such self-organizing neural network can make effective use of domain knowledge to improve learning efficiency and reduce model complexity.
Keywords
Adaptive resonance theory (ART), domain knowledge, reinforcement learning (RL), self-organizing neural networks
Discipline
Computer and Systems Architecture | Databases and Information Systems | OS and Networks
Research Areas
Data Science and Engineering
Publication
IEEE Transactions on Neural Networks and Learning Systems
Volume
26
Issue
5
First Page
889
Last Page
902
ISSN
2162-2388
Identifier
10.1109/TNNLS.2014.2327636
Publisher
Institute of Electrical and Electronics Engineers
Citation
TENG, Teck-Hou; TAN, Ah-hwee; and ZURADA, Jacek M..
Self-organizing neural networks integrating domain knowledge and reinforcement learning. (2015). IEEE Transactions on Neural Networks and Learning Systems. 26, (5), 889-902.
Available at: https://ink.library.smu.edu.sg/sis_research/5236
Copyright Owner and License
Authors
Creative Commons License
This work is licensed under a Creative Commons Attribution-NonCommercial-No Derivative Works 4.0 International License.
Additional URL
https://doi.org/10.1109/TNNLS.2014.2327636
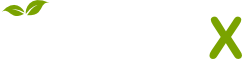
- Citations
- Citation Indexes: 44
- Usage
- Downloads: 127
- Abstract Views: 29
- Captures
- Readers: 97
- Mentions
- References: 1
Included in
Computer and Systems Architecture Commons, Databases and Information Systems Commons, OS and Networks Commons