Publication Type
Conference Proceeding Article
Version
publishedVersion
Publication Date
6-2019
Abstract
Meta-learning has been proposed as a framework to address the challenging few-shot learning setting. The key idea is to leverage a large number of similar few-shot tasks in order to learn how to adapt a base-learner to a new task for which only a few labeled samples are available. As deep neural networks (DNNs) tend to overfit using a few samples only, meta-learning typically uses shallow neural networks (SNNs), thus limiting its effectiveness. In this paper we propose a novel few-shot learning method called meta-transfer learning (MTL) which learns to adapt a deep NN for few shot learning tasks. Specifically, "meta" refers to training multiple tasks, and "transfer" is achieved by learning scaling and shifting functions of DNN weights for each task. In addition, we introduce the hard task (HT) meta-batch scheme as an effective learning curriculum for MTL. We conduct experiments using (5-class, 1-shot) and (5-class, 5-shot) recognition tasks on two challenging few-shot learning benchmarks: miniImageNet and Fewshot-CIFAR100. Extensive comparisons to related works validate that our meta-transfer learning approach trained with the proposed HT meta-batch scheme achieves top performance. An ablation study also shows that both components contribute to fast convergence and high accuracy.
Keywords
Few-shot learning, meta-learning, hard task mining, object classification
Discipline
Artificial Intelligence and Robotics | Numerical Analysis and Scientific Computing
Research Areas
Data Science and Engineering
Publication
2019 IEEE/CVF Conference on Computer Vision and Pattern Recognition: Long Beach, CA, June 16-20: Proceedings
First Page
403
Last Page
412
ISBN
9781728132938
Identifier
10.1109/CVPR.2019.00049
Publisher
IEEE Computer Society
City or Country
Los Alamitos, CA
Citation
SUN, Qianru; LIU, Yaoyao; CHUA, Tat-Seng; and SCHIELE, Bernt.
Meta-transfer learning for few-shot learning. (2019). 2019 IEEE/CVF Conference on Computer Vision and Pattern Recognition: Long Beach, CA, June 16-20: Proceedings. 403-412.
Available at: https://ink.library.smu.edu.sg/sis_research/4447
Copyright Owner and License
Authors
Creative Commons License
This work is licensed under a Creative Commons Attribution-NonCommercial-No Derivative Works 4.0 International License.
Additional URL
https://doi.org/10.1109/CVPR.2019.00049
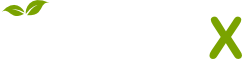
- Citations
- Citation Indexes: 1086
- Usage
- Downloads: 456
- Abstract Views: 247
- Captures
- Readers: 1213
Included in
Artificial Intelligence and Robotics Commons, Numerical Analysis and Scientific Computing Commons