Publication Type
Conference Proceeding Article
Version
publishedVersion
Publication Date
8-2017
Abstract
Object detection in images is a crucial task in computer vision, with important applications ranging from security surveillance to autonomous vehicles. Existing state-of-the-art algorithms, including deep neural networks, only focus on utilizing features within an image itself, largely neglecting the vast amount of background knowledge about the real world. In this paper, we propose a novel framework of knowledge-aware object detection, which enables the integration of external knowledge such as knowledge graphs into any object detection algorithm. The framework employs the notion of semantic consistency to quantify and generalize knowledge, which improves object detection through a re-optimization process to achieve better consistency with background knowledge. Finally, empirical evaluation on two benchmark datasets show that our approach can significantly increase recall by up to 6.3 points without compromising mean average precision, when compared to the state-of-the-art baseline.
Keywords
Machine Learning, Knowledge-based Learning, Robotics and Vision, Vision and Perception, Artificial intelligence, Deep neural networks, Object recognition, Optimization, Semantics
Discipline
Databases and Information Systems | Graphics and Human Computer Interfaces | Theory and Algorithms
Research Areas
Data Science and Engineering
Publication
Proceedings of the Twenty-Sixth International Joint Conference on Artificial Intelligence: Melbourne, Australia, August 19-25
First Page
1661
Last Page
1667
ISBN
9780999241103
Identifier
10.24963/ijcai.2017/230
Publisher
International Joint Conferences on Artificial Intelligence
City or Country
Melbourne
Citation
FANG, Yuan; KUAN, Kingsley; LIN, Jie; TAN, Cheston; and CHANDRASEKHAR, Vijay.
Object detection meets knowledge graphs. (2017). Proceedings of the Twenty-Sixth International Joint Conference on Artificial Intelligence: Melbourne, Australia, August 19-25. 1661-1667.
Available at: https://ink.library.smu.edu.sg/sis_research/4067
Creative Commons License
This work is licensed under a Creative Commons Attribution-NonCommercial-No Derivative Works 4.0 International License.
Additional URL
https://doi.org/10.24963/ijcai.2017/230
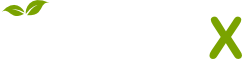
- Citations
- Patent Family Citations: 1
- Citation Indexes: 95
- Usage
- Downloads: 1198
- Abstract Views: 734
- Captures
- Readers: 141
Included in
Databases and Information Systems Commons, Graphics and Human Computer Interfaces Commons, Theory and Algorithms Commons