Publication Type
Journal Article
Version
acceptedVersion
Publication Date
10-2018
Abstract
The primary goal of Automated Program Repair (APR) is to automatically fix buggy software, to reduce the manual bug-fix burden that presently rests on human developers. Existing APR techniques can be generally divided into two families: semantics- vs. heuristics-based. Semantics-based APR uses symbolic execution and test suites to extract semantic constraints, and uses program synthesis to synthesize repairs that satisfy the extracted constraints. Heuristic-based APR generates large populations of repair candidates via source manipulation, and searches for the best among them. Both families largely rely on a primary assumption that a program is correctly patched if the generated patch leads the program to pass all provided test cases. Patch correctness is thus an especially pressing concern. A repair technique may generate overfitting patches, which lead a program to pass all existing test cases, but fails to generalize beyond them. In this work, we revisit the overfitting problem with a focus on semantics-based APR techniques, complementing previous studies of the overfitting problem in heuristics-based APR. We perform our study using IntroClass and Codeflaws benchmarks, two datasets well-suited for assessing repair quality, to systematically characterize and understand the nature of overfitting in semantics-based APR. We find that similar to heuristics-based APR, overfitting also occurs in semantics-based APR in various different ways.
Keywords
Automated program repair, Program synthesis, Symbolic execution, Patch overfitting
Discipline
Software Engineering
Research Areas
Software and Cyber-Physical Systems
Publication
Empirical Software Engineering
Volume
23
Issue
5
First Page
3007
Last Page
3033
ISSN
1382-3256
Identifier
10.1007/s10664-017-9577-2
Publisher
Springer Verlag (Germany)
Citation
LE, Dinh Xuan Bach; THUNG, Ferdian; LO, David; and LE GOUES, Claire.
Overfitting in semantics-based automated program repair. (2018). Empirical Software Engineering. 23, (5), 3007-3033.
Available at: https://ink.library.smu.edu.sg/sis_research/3986
Copyright Owner and License
Authors
Creative Commons License
This work is licensed under a Creative Commons Attribution-NonCommercial-No Derivative Works 4.0 International License.
Additional URL
https://doi.org/10.1007/s10664-017-9577-2
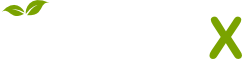
- Citations
- Citation Indexes: 63
- Usage
- Downloads: 409
- Abstract Views: 58
- Captures
- Readers: 63
- Mentions
- References: 1