Publication Type
Conference Proceeding Article
Version
acceptedVersion
Publication Date
12-2014
Abstract
Several automated parameter tuning procedures/configurators have been proposed in order to find the best parameter setting for a target algorithm. These configurators can generally be classified into model-free and model-based approaches. We introduce a recent approach which is based on the hybridization of both approaches. It combines the Design of Experiments (DOE) and Response Surface Methodology (RSM) with prevailing model-free techniques. DOE is mainly used for determining the importance of parameters. A First Order-RSM is initially employed to define the promising region for the important parameters. A Second Order-RSM is then built to approximate the center point as well as the final promising ranges of parameter values. We show how our approach can be embedded with existing model-free techniques, namely ParamILS and Randomized Convex Search, to tune target algorithms and demonstrate that our proposed methodology leads to improvements in terms of the quality of the solutions compared against the earlier work.
Keywords
Design of Experiment, Parameter Tuning, Response Surface Methodology, Second Order Model
Discipline
Artificial Intelligence and Robotics | Computer Sciences | Operations Research, Systems Engineering and Industrial Engineering
Research Areas
Intelligent Systems and Optimization
Publication
2014 IEEE International Conference on Industrial Engineering and Engineering Management (IEEM): 9-12 December, Selangor: Proceedings
First Page
652
Last Page
656
ISBN
9781479964109
Identifier
10.1109/IEEM.2014.7058719
Publisher
IEEE
City or Country
Piscataway, NJ
Citation
GUNAWAN, Aldy and LAU, Hoong Chuin.
Second Order-Response Surface Model for the Automated Parameter Tuning Problem. (2014). 2014 IEEE International Conference on Industrial Engineering and Engineering Management (IEEM): 9-12 December, Selangor: Proceedings. 652-656.
Available at: https://ink.library.smu.edu.sg/sis_research/2672
Copyright Owner and License
Authors/LARC
Creative Commons License
This work is licensed under a Creative Commons Attribution-NonCommercial-No Derivative Works 4.0 International License.
Additional URL
https://doi.org/10.1109/IEEM.2014.7058719
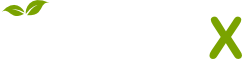
- Citations
- Citation Indexes: 3
- Patent Family Citations: 1
- Usage
- Downloads: 1266
- Abstract Views: 17
- Captures
- Readers: 9
Included in
Artificial Intelligence and Robotics Commons, Operations Research, Systems Engineering and Industrial Engineering Commons