Publication Type
Conference Proceeding Article
Version
acceptedVersion
Publication Date
10-2020
Abstract
Despite significant advances in deep learning based object detection in recent years, training effective detectors in a small data regime remains an open challenge. This is very important since labelling training data for object detection is often very expensive and time-consuming. In this paper, we investigate the problem of few-shot object detection, where a detector has access to only limited amounts of annotated data. Based on the meta-learning principle, we propose a new meta-learning framework for object detection named "Meta-RCNN", which learns the ability to perform few-shot detection via meta-learning. Specifically, Meta-RCNN learns an object detector in an episodic learning paradigm on the (meta) training data. This learning scheme helps acquire a prior which enables Meta-RCNN to do few-shot detection on novel tasks. Built on top of the popular Faster RCNN detector, in Meta-RCNN, both the Region Proposal Network (RPN) and the object classification branch are meta-learned. The meta-trained RPN learns to provide class-specific proposals, while the object classifier learns to do few-shot classification. The novel loss objectives and learning strategy of Meta-RCNN can be trained in an end-to-end manner. We demonstrate the effectiveness of Meta-RCNN in few-shot detection on three datasets (Pascal-VOC, ImageNet-LOC and MSCOCO) with promising results.
Keywords
Object Detection, Deep Learning, Meta Learning
Discipline
Databases and Information Systems | Data Science
Research Areas
Data Science and Engineering
Areas of Excellence
Digital transformation
Publication
MM '20: Proceedings of the 28th ACM International Conference on Multimedia, Seattle, WA, USA, October 12-16
First Page
1679
Last Page
1687
Identifier
10.1145/3394171.3413832
Publisher
ACM
City or Country
New York
Embargo Period
2-10-2025
Citation
WU, Xiongwei; SAHOO, Doyen; and HOI, Steven.
Meta-RCNN: Meta learning for few-shot object detection. (2020). MM '20: Proceedings of the 28th ACM International Conference on Multimedia, Seattle, WA, USA, October 12-16. 1679-1687.
Available at: https://ink.library.smu.edu.sg/sis_research/10097
Creative Commons License
This work is licensed under a Creative Commons Attribution-NonCommercial-No Derivative Works 4.0 International License.
Additional URL
https://doi.org/10.1145/3394171.3413832
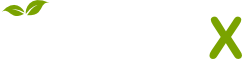
- Citations
- Citation Indexes: 69
- Patent Family Citations: 1
- Usage
- Downloads: 12
- Abstract Views: 1
- Captures
- Readers: 62