Publication Type
Conference Proceeding Article
Version
publishedVersion
Publication Date
4-2018
Abstract
As malicious behaviors vary significantly across mobile malware, it is challenging to detect malware both efficiently and effectively. Also due to the continuous evolution of malicious behaviors, it is difficult to extract features by laborious human feature engineering and keep up with the speed of malware evolution. To solve these challenges, we propose DeepRefiner to identify malware both efficiently and effectively. The novel technique enabling effectiveness is the semantic-based deep learning. We use Long Short Term Memory on the semantic structure of Android bytecode, avoiding missing the details of method-level bytecode semantics. To achieve efficiency, we apply Multilayer Perceptron on the xml files based on the finding that most malware can be efficiently identified using information only from xml files. We evaluate the detection performance of DeepRefiner with 62,915 malicious applications and 47,525 benign applications, showing that DeepRefiner effectively detects malware with an accuracy of 97.74% and a false positive rate of 2.54%. We compare DeepRefiner with a state-of-the-art single classifierbased detection system, StormDroid, and ten widely used signature-based anti-virus scanners. The experimental results show that DeepRefiner significantly outperforms StormDroid and anti-virus scanners. In addition, we evaluate the robustness of DeepRefiner against typical obfuscation techniques and adversarial samples. The experimental results demonstrate that DeepRefiner is robust in detecting obfuscated malicious applications.
Keywords
Deep Neural Networks, Malware Detection, Mobile Security
Discipline
Information Security
Research Areas
Cybersecurity
Publication
2018 IEEE European Symposium on Security and Privacy Workshops (EUROS&P) 2018: April 24-26, London: Proceedings
First Page
473
Last Page
487
ISBN
9781538642276
Identifier
10.1109/EuroSP.2018.00040
Publisher
IEEE
City or Country
Piscataway, NJ
Embargo Period
2-10-2025
Citation
KE, Xu; Li, Yingjiu; DENG, Robert H.; and CHEN, Kai.
DeepRefiner: Multi-layer Android malware detection system applying deep neural networks. (2018). 2018 IEEE European Symposium on Security and Privacy Workshops (EUROS&P) 2018: April 24-26, London: Proceedings. 473-487.
Available at: https://ink.library.smu.edu.sg/sis_research/10094
Creative Commons License
This work is licensed under a Creative Commons Attribution-NonCommercial-No Derivative Works 4.0 International License.
Additional URL
https://doi.org/10.1109/EuroSP.2018.00040
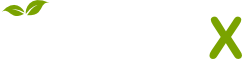
- Citations
- Citation Indexes: 123
- Patent Family Citations: 1
- Usage
- Abstract Views: 172
- Downloads: 28
- Captures
- Readers: 132