Publication Type
Journal Article
Version
submittedVersion
Publication Date
7-2023
Abstract
Problem definition: Shared micromobility vehicles provide an eco-friendly form of short-distance travel within an urban area. Since customers pick up and drop off vehicles in any service region at any time, such convenience often leads to a severe imbalance between vehicle supply and demand in different service regions. To overcome this, a micromobility operator can crowdsource individual riders with reward incentives in addition to engaging a third-party logistics provider (3PL) to relocate the vehicles. Methodology/results: We construct a time-space network with multiple service regions and formulate a twostage stochastic mixed-integer program considering uncertain customer demands. In the first stage, the operator decides the initial vehicle allocation for the regions, whereas in the second stage, he determines subsequent vehicle relocation across the regions over an operational horizon. We develop an efficient solution approach that incorporates scenario-based and time-based decomposition techniques. Our approach outperforms a commercial solver in solution quality and computational time for solving large-scale problem instances based on real data. Managerial implications: The budgets for acquiring vehicles and for rider crowdsourcing significantly impact the vehicle initial allocation and subsequent relocation. Introducing rider crowdsourcing in addition to the 3PL can significantly increase profit, reduce demand loss, and improve the vehicle utilization rate of the system without affecting any existing commitment with the 3PL. The 3PL is more efficient for mass relocation than rider crowdsourcing, while the latter is more efficient in handling sporadic relocation needs. To serve a region, the 3PL often relocates vehicles in batches from faraway, low-demand regions around peak hours of a day, whereas rider crowdsourcing relocates a few vehicles each time from neighboring regions throughout the day. Furthermore, rider crowdsourcing relocates more vehicles under a unimodal customer arrival pattern than a bimodal pattern, whereas the reverse holds for the 3PL.
Keywords
Shared Micromobility, Crowdsourcing, Allocation and Relocation, Two-stage Stochastic Mixed-integer Programming, Decomposition Algorithm
Discipline
Operations and Supply Chain Management | Transportation
Research Areas
Operations Management
Publication
Manufacturing & Service Operations Management
Volume
25
Issue
4
First Page
1394
Last Page
1415
ISSN
1523-4614
Identifier
10.1287/msom.2023.1199
Publisher
Institute for Operations Research and Management Sciences
Citation
JIN, Ziliang; WANG, Yulan; LIM, Yun Fong; PAN, Kai; and SHEN, Zuo-Jun Max.
Vehicle rebalancing in a shared micromobility system with rider crowdsourcing. (2023). Manufacturing & Service Operations Management. 25, (4), 1394-1415.
Available at: https://ink.library.smu.edu.sg/lkcsb_research/7175
Copyright Owner and License
Authors
Creative Commons License
This work is licensed under a Creative Commons Attribution-NonCommercial-No Derivative Works 4.0 International License.
Additional URL
https://doi.org/10.1287/msom.2023.1199
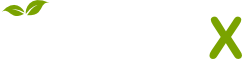
- Citations
- Citation Indexes: 8
- Usage
- Downloads: 481
- Abstract Views: 48
- Captures
- Readers: 36
- Mentions
- News Mentions: 1