Publication Type
Journal Article
Version
acceptedVersion
Publication Date
8-2014
Abstract
In this paper we propose a revised version of (bagging) bootstrap aggregating as a forecast combination method for the out-of-sample forecasts in time series models. The revised version explicitly takes into account the dependence in time series data and can be used to justify the validity of bagging in the reduction of mean squared forecast error when compared with the unbagged forecasts. Monte Carlo simulations show that the new method works quite well and outperforms the traditional one-step-ahead linear forecast as well as the nonparametric forecast in general, especially when the in-sample estimation period is small. We also find that the bagging forecasts based on misspecified linear models may work as effectively as those based on nonparametric models, suggesting the robustification property of bagging method in terms of out-of-sample forecasts. We then reexamine forecasting powers of predictive variables suggested in the literature to forecast the excess returns or equity premium. We find that, consistent with Goyal and Welch (2008), the historical average excess stock return forecasts may beat other predictor variables in the literature when we apply traditional one-step linear forecast and the nonparametric forecasting methods. However, when using the bagging method or its revised version, which help to improve the mean squared forecast error for unstable predictors, the predictive variables have a better forecasting power than the historical mean.
Keywords
Bagging, Combined forecasts, Nonparametric Models, Predictability, Time Series
Discipline
Econometrics
Research Areas
Econometrics
Publication
Econometric Reviews
Volume
33
Issue
5-6
First Page
575
Last Page
605
ISSN
0747- 4938
Identifier
10.1080/07474938.2013.825142
Publisher
Taylor and Francis
Citation
JIN, Sainan; SU, Liangjun; and ULLAH, Aman.
Robustify Financial Time Series Forecasting with Bagging. (2014). Econometric Reviews. 33, (5-6), 575-605.
Available at: https://ink.library.smu.edu.sg/soe_research/1428
Copyright Owner and License
Authors
Creative Commons License
This work is licensed under a Creative Commons Attribution-NonCommercial-No Derivative Works 4.0 International License.
Additional URL
https://doi.org/10.1080/07474938.2013.825142
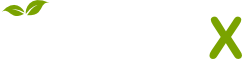
- Citations
- Citation Indexes: 23
- Policy Citations: 4
- Usage
- Downloads: 975
- Abstract Views: 239
- Captures
- Readers: 36