Publication Type
Journal Article
Publication Date
3-1995
Abstract
Global energy models have a large degree of uncertainty associated with them. This consists of uncertainty in the model structure as well as uncertainty in the exogenous input parameters. This paper combines Monte Carlo methods with Bayesian updating techniques to provide a method for refining the uncertainty in the Edmonds-Reilly global energy model. The Bayesian updating technique uses likelihood-based windows constructed from actual observations of the output variables to filter out the model simulations that do not conform with the observed output. The windows are based on outputs of energy consumption and carbon emissions. Two alternative model structures are examined: one with uncorrelated input parameters and the other with correlated input parameters. Statistical properties are calculated to measure the effects of windowing on the output distributions. The partial rank correlations between the inputs and outputs and between the inputs are also determined. The prior distributions and correlation structure of the inputs are then revised through the updating process. An application of the windowing process illustrates the effects of capping carbon emissions on the input structure.
Keywords
Bayesian updating, Forecasting, Energy model, Uncertainty analysis
Discipline
Business | Management Sciences and Quantitative Methods
Research Areas
Strategy and Organisation
Publication
International Journal of Forecasting
Volume
11
Issue
1
First Page
43
Last Page
61
ISSN
0169-2070
Identifier
10.1016/0169-2070(94)02010-M
Publisher
Elsevier
Citation
Tschang, F. Ted and Dowlatabadi, Hadi.
A Bayesian technique for refining the uncertainty in global energy model forecasts. (1995). International Journal of Forecasting. 11, (1), 43-61.
Available at: https://ink.library.smu.edu.sg/lkcsb_research/2283
Copyright Owner and License
Publisher
Creative Commons License
This work is licensed under a Creative Commons Attribution-NonCommercial-No Derivative Works 4.0 International License.
Additional URL
https://doi.org/10.1016/0169-2070(94)02010-M
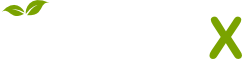
- Citations
- Citation Indexes: 15
- Policy Citations: 1
- Usage
- Abstract Views: 16
- Downloads: 14
- Captures
- Readers: 24